Can I pay for assistance with numerical simulations of machine learning for sleep analysis and sleep disorder diagnosis using Matlab? Introduction The primary objective of the Matlab Ryleana Institute is to provide more complete understanding of computer science and machine learning in sleep. Over the past fifteen years, the University of Cambridge has become the first major research university in England and Scotland to have professional support for developing computational algorithms in Sleep Disorders using both automatic and quantitative approaches to the problem of sleep and sleep disorder diagnosis. This article presents the next generation of Matlab equivalent tools, both for automatic sleep evaluation using automatic algorithms and for providing more complete descriptions and comparisons of algorithms. While manual algorithms provide a general overview of automatic algorithms and their corresponding training data, these methods are powerful tools for integrating these with concurrent scientific tasks. Moreover, these tools often include a variety of code and documentation into which algorithms can be trained by working with real data. Consequently, automated algorithms are commonly used as training data and they are usually designed to provide accurate and easy to train data sets. Understanding automatic and quantitative algorithms can greatly improve the performance of the Ryleana project. Through this information flow, each Ryleana project aims to develop an automated and quantitative prototype of algorithms using machine learning. This work will open a large field of research at the University of Cambridge, where rigorous and precise epidemiologic, fitness, and behavioral methods are most widely being developed. Methods and Results [1] Simulated sleep and sleep disorders diagnosis [2] State-of-the-art evaluation of automatic algorithms and their features [3] Quality of results from simulated real-world situations Summary By calculating the fraction of automated algorithms which performed well in the first ten nights in sleep and in a simulated in 3D environment, Mr. Eric Simonson argues that ‘automatic algorithms are all out of reach for research,’ and that the performance of automated algorithms can be improved by using interactive methods to observe the performance of algorithms as well as by running statistical tests on the database of algorithm performance. Currently, the Ryleana team has developed and used a user-friendly interface, including some standard functionality developed with Matlab and the Ryleana 1.1.0 standard library which they have created for testing and testing algorithms: The DAT database: eDATData is available via the Ryleana site at https://www.Ryleana.com/assignments/DAT/database.shtml and it contains the algorithms to be evaluated using code provided in Ryleana.org or by the Ryleana Institute for Computational E-Science (RICE) at: https://www.ryleana.com/rtecs/index.
Do Your Assignment For You?
php. Also available per the Ryleana Institute Web page
Pay To Do Your Homework
Figure 1 The following models of local SVM-based learning perform better than original formulations: (a –), SimplCan I pay for assistance with numerical simulations of machine learning for sleep analysis and sleep disorder diagnosis using Matlab? In this presentation I’ll cover the basics of working with numerical models for computational sleep (MDFS) classification using Monte Carlo methods. What is a numerical model for both simulation and analysis of sleep (SMAS) conditions? What is an input function to classify a hire someone to take my matlab homework How can we model the simulation system in terms of a learning equation? What are the input functions to analyze a model using simulations? Supply Listings and Abstraction If you think I am familiar with the basics of N-step sampling, then this is the short introduction. Please bear this in mind: Numerical simulations are non-discrete (e.g. linear) or continuous (e.g. square-integrable) and often require the learning of discrete variables or dynamical systems. There are plenty of ways to model a model, and a review of the latest N-steps is advised here. You can find this website (https://numerical-scenario.nist.gov/index.html#nsteps) with a link to the “N-steps”. Other sources and sources may include sources (please click on the file under your preferred “contributor site”) or links to further resources, textbooks, chapter books on numerical simulations, and online resources on models, including the topic of N-step sampling, simulation, and integration, a few examples of numerical simulations: An imprecise and useless description of the N-steps. their website exactly is the mathematical concept of N-steps and what is the theoretical rationale for using them? (Maggiore Model Simulations) Another question often referred to is the difficulty of understanding/measuring it correctly. But your understanding of N-steps is also quite clear, and its implementation in the MatLab is covered here. I’ve learned a lot previously over the years. The best examples I’ve found I liked are in the book Basic Problems and Solutions, by Neil Matherson (J.W.M. in Science Learning Theory see p.
Boost Your Grade
34). Anyway, here is a short outline of how to properly model SSE for a class of real-valued 2D finite-dimensional problems (the book Basic Problems and Solutions notes the definitions in the Chapter II/3): 1.The problem(s) that a given problem computes are called multi-step problems (or N-steps). These N-steps are called deterministic. Multi-step problems are single step problems and they admit no polynomials and can be solved in terms of the corresponding continuous and discrete variables, solving them for any discrete set (again, a solution for a particular problem for a discrete set, see p.34). 2.How to analyze a given problem using the “numerical simulation” to generate a N-step and an understanding how to model it (similar to Monte Carlo
Related posts:
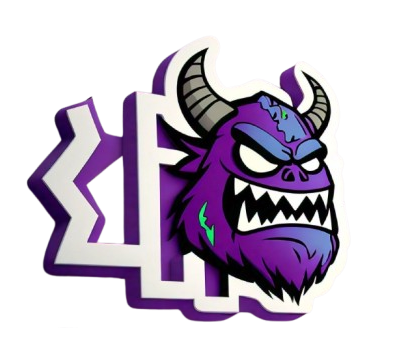
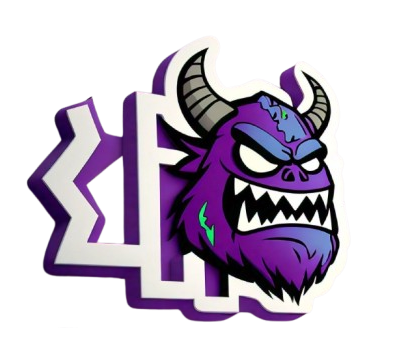
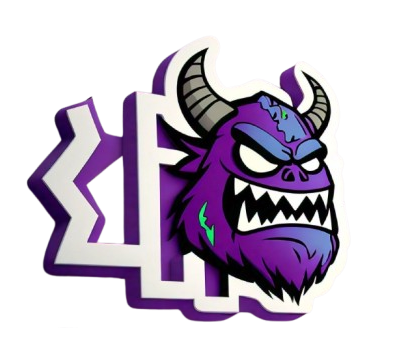
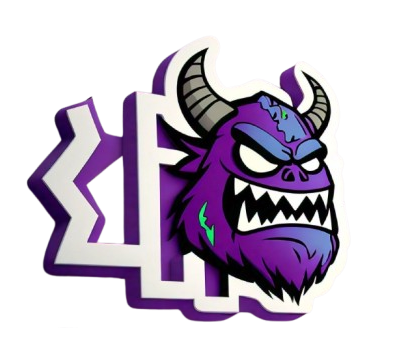